What is Data-Driven Agriculture?
Data-driven agriculture means maximizing insights from your data toward your return on investment in a sustainable approach. This means to make the best with your data considering the uncertainties ahead. Agricultural activities and specifically farming are complex processes with a high number of variables involved. Thanks to advances in precision agriculture, different types of data are collected from the farms. A data driven approach provide farmers and growers actionable intelligence extracted from the data toward optimizing their practices.
How is Data Used in Agriculture?
However, ‘Having all other factors unchanged’ is an assumption that never happens in farming. In reality, these variables change simultaneously and each one is affected by others. Rain and irrigation could wash down nitrogen and herbicide. Nitrogen and weeds affect the uptake of water by plants. Weeds consume part of nitrogen out of soil, and nitrogen affects the efficiency of herbicide. There are many more factors involved in farming, and as a result, data-driven decision is not a straightforward task even having all the data collected.
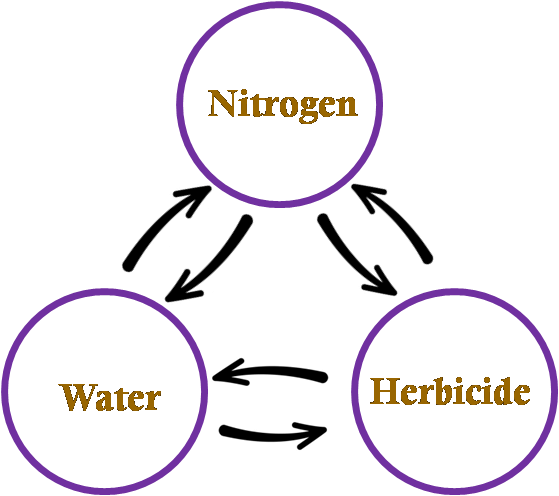
How does AI Help in Data-Driven Agriculture?
Generative AI is the talk of the town, a stark contrast to its quieter reception in 2021. Dive into our latest post exploring the challenges and opportunities presented by large language models such as GPT in agriculture.
Although complexity in data can overwhelm the human mind, AI algorithms are structured to deal with it effectively. These algorithms are designed to find hidden patterns in data and predict outcomes, which leads to data-driven decisions that are proactive rather than reactive. Training a reliable machine learning model is at the heart of any complex data-driven agriculture. This leads to the next phase which is translating the insight from artificial intelligence to an actionable intelligence where decisions can be made upon it.
A Case-Study on Application of Data-Driven Farming
Let say we inform the farmer that he or she should expect a slight occurrence of foliar diseases in the coming weeks. Based on this new piece of information, the farmer evaluates whether or not they should pay for fungicides this farming season.
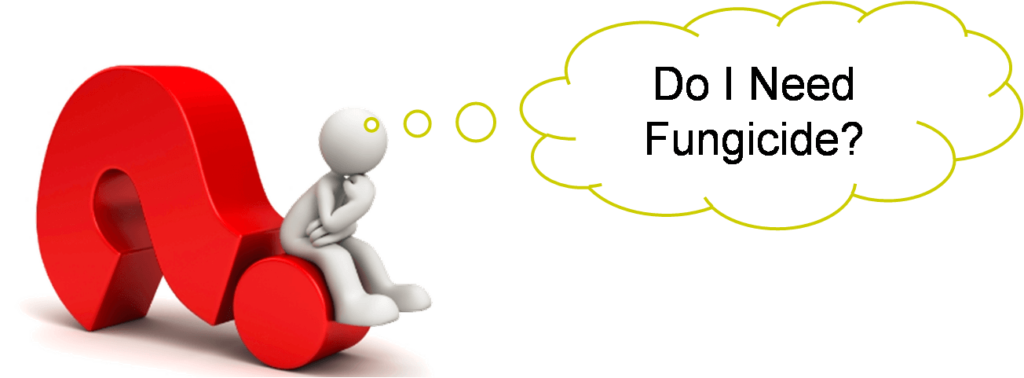
This is where data-driven decision takes place. It is to make the best of the information you have even though there is uncertainty around that. One side of the equation is that the costs associated with purchasing and applying fungicides. The other side of the equation is the yield loss because of an occurrence of foliar diseases. This can be estimated from historical data, multiplied by dollar value of that loss, multiplied by how likely is that to happen, which comes from machine learning model. Comparing two sides of this equation leads to an informed decision.
This was a simple example and farmers need to evaluate more factors before making decisions, but it shows how AI and data driven approach could provide farmers with another piece of information to help them make the best decisions.
There is a gap between extensive data collected from farms and its usability for the farmers. The complexity of agricultural problems cannot be addressed fully by experts where data is too big for human mind to digest. We help farmers and agronomists to better make sense of their vast data and add to their expertise.